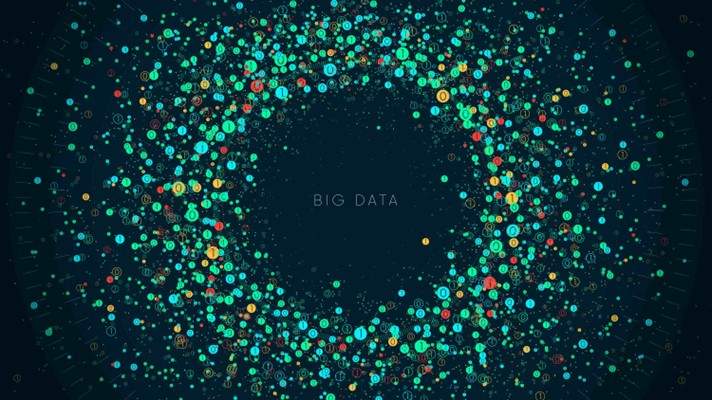
The financial services sector is one of the most data-intensive sectors in the global economy. Here, we discuss the impact of big data in banking, including personal banking, business banking, and investment banking. Read on to learn the role of big data in each of these areas and how Xtracta’s automatic data extraction technology and OCR software enable financial companies to best use big data to their advantage.
What Does the Term ‘Banking’ Encompass?
The term ‘banking’ is a term that encompasses a wide range of areas. Banking is very data dependent. For example, personal credit (credit cards, home loans, car loans, etc.) involves a very small-scale set of data that indicates how a person runs their financial lives, like whether they can service a loan and whether they should obtain one in the first place.
On the opposite end of the spectrum exists the complex world of investment banking. Here, considerable data is necessary to determine trends: how prices for the likes of shares may fluctuate, different industry trends, and more. The blanket term ‘banking’ encompasses all these areas.
Many people think of banking as simply incoming (paycheques) and outgoing (bills and groceries) money but banking—especially investment banking—is far more complicated.
Below, we separate the concept of big data in banking into three different categories. Learn the differences between big data in personal banking, business banking, and investment banking here.
Big Data in Personal Banking
For personal banking, banks must ensure that people can make their repayments when giving out credit. They may look at big data and some personal attributes to determine this. Creditworthiness, for both people and businesses, is often determined by the five Cs.
- Character: a borrower’s integrity (determined by credit checks, assets/savings relative to age, occupation and stability, bank statements-spending patterns, etc.)
- Capacity to Repay: the applicant’s ability to repay the loan
- Collateral: assets to secure a loan
- Capital: the amount of deposit or equity of the borrower/applicant
- Conditions: the economic health of the market
These general principles help to create a solid credit analysis framework and determine a person’s risk. For example, an older adult who has either worked at a job for many years, not changed their career, or is in a very reliable profession (e.g., accounting), has a good chance of getting credit. In comparison, a young adult working at McDonald’s (typically a transitory job) is less likely to receive credit.
With data and tools like Xtracta more readily available these days, lending decisions are becoming more granular. Rather than focusing on very high-level attributes of a potential borrower, there’s also a lot more data that banks can use to determine an individual’s creditworthiness. For example:
- Specific behaviours (e.g., analysing the spending on their credit cards or debit cards),
- Consistency of income,
- Education profiles,
- Etc.
Based on that information, they can determine whether to give a loan. In what areas can Xtracta help? We can assist in the transfer and receipt of bank data. For example, a person wants to borrow money to buy a house from bank A. If all their transaction histories are in bank B, bank A may wish to obtain that transaction history from Bank B to analyse it. Traditionally, this data would be available in paper or PDF form. With an automated data extraction system like Xtracta, bank A can pull that information directly from the paper or PDF documents from bank B.
Big Data in Business Banking
Traditionally in business banking, banks may look at higher-end trends in an industry (general economic trends) to determine a business’s creditworthiness. For example, a company in an out-of-fashion industry like the phonebook printing industry are likely not in a growth industry, and there is a general trend for the industry to be declining. While this affects their creditworthiness, companies in fading industries may have good profit margins, showing them as reliable for banks to lend to.
Banks consider more than just higher-end trends to determine a company’s creditworthiness. Companies operate individually, and just because a given industry works in a certain way doesn’t mean a business within that industry will follow suit. A current industry example of this is the construction sector. While the overall industry is booming, many construction companies struggle because of poor business management. A company in that industry may struggle often for various reasons including:
- It doesn’t ensure adequate cash is collected consistently from customers, or
- It has contractors with payment terms that are too short for them to cover.
From a perspective of lending against these companies, a bank needs to look at how they run their business individually to determine whether giving them business loans is a good idea.
How do banks determine whether a business is creditworthy? One way is to look at a company’s financial statements. A company with a large accounts receivable—compared to other companies of similar size—indicates the company is bad at collections. Contrastingly, if a company’s accounts payable is large compared to others in the industry, this may reflect positively on them, showing they have extended credit terms with their suppliers.
Traditionally this kind of information is locked up in a company’s financial reports but as more people move towards new-age accounting software, this data becomes more accessible for banks. However, for many older businesses or those that have yet to incorporate this new technology, they must produce reports like profit and loss statements, balance sheets, etc., to send to the bank for review.
Investing in technology like Xtracta is an opportunity for banks to pull this data from such reports and feed it into systems that can automatically determine how a business is performing compared to peers in the industry and thus, determine whether they should give credit.
Big Data in Investment Banking
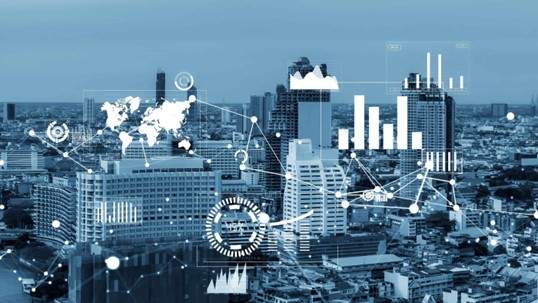
Investment banking requires the use of some of the most complex financial mechanisms in the world. Unique to personal banking and business banking, investment banking is a division that serves many different purposes, focusing on creating capital for governments, companies, and other entities. Considered big gambling, an example of investment banking in recent history is the 2008 financial crisis (a.k.a., ‘The Great Recession’), caused by investment banks making subprime loans and trading risky securities.
Those taking part in investment banking today look for the top trends shaping industries and other macro-economic factors. Investment banks are conducting huge amounts of data analysis to determine where they think the markets will move next, as the slightest update in data can impact their forecast. Big data is critical in this area of banking. Investors need machine learning models to perform analyses almost in real-time so that trades can take place faster than their competitors.
Big Data and Investing in Financial Products
To explore this further, let’s investigate how financial products work in investment banking. In general terms, financial products are contracts bought and sold with others on a market. In investment banking, the financial product you buy might be a ‘future’, e.g., what you predict the price of oil to be in 12 months. This move is a gamble on your expectation of where the market is going. Banks will trade those futures depending on what they feel the real value is and the accuracy of the financial product.
When setting expectations of where the market price for oil is going, a wide range of analytics needs to be considered. For example, global issues like the war in Ukraine drastically affect these futures. Within the first minute (or even less) that news of the invasion was heard, the value of oil and the value of oil futures increased dramatically. This is because the obvious conclusions of a war in Ukraine are sanctions on Russia (a major oil producer), disruptions to supply pipelines, etc. In this case, those ‘futures’ become more valuable. People who already have them will want to keep them, and those who don’t have them will want to try to buy them before the rest of the market moves in and the prices increase.
Here, big data is critical because investors must be able to predict this or have models that can show what will happen based on big data. These models, built from big data, also need to be constantly fed new input data, and this needs to happen faster than humanly possible (e.g., real-time analytics from news streams).
This is one side of investment banking, and as we have demonstrated, big data is essential to build those models, analyse the news, and make trades. Where Xtracta gets involved is often around how parties interact.
For example, we’ve worked with trading parties (large investment banks) who required contracts with other banks and financial institutions governing how they trade, collateral that gets posted etc. By integrating Xtracta’s contract capture API, these banks could streamline processing to receive the data from the counterparties and fill that data into their computer systems.
Much of contract analysis involves complex semi-structured data and pulling out pertinent information from it. Xtracta facilitates this process, enabling data transferral and processing between investment banks, making it easier for them to trade and feed relevant information into their systems.
The Future of Banking and Where Xtracta Fits In
The industry is moving towards more digitisation, especially for consumer and business banking. We’ve worked with banks interested in doing real-time loan approvals through chatbots (e.g., through Facebook Messenger), where applicants simply drop and upload all their documents into the chat for review. A couple of minutes later, the chatbot—using Xtracta’s technology to automatically capture data from the uploaded documents—analyses and pre-approves or declines the credit application.
Compared to traditional banking where you must fill in forms, send them away, and then maybe get a ‘yes’ or a ‘no’ a couple of weeks later, modern technological advancements like Xtracta are changing this process along with the consumer and business expectations towards it.
Nowadays, consumer and business expectations around credit and loan applications are for almost immediate decision-making from banks. Therefore, access to big data and models to generate results is critical. Technology like Xtracta is also critical for lenders, as it allows them to receive client information and make decisions quickly. For lenders that are not implementing this type of technology, there is a high chance of being left in the dust of their competitors.
Get in Touch to Discuss the Possibilities of Implementing Xtracta in Your Organisation
The possibilities of AI and machine learning are endless. If you’re interested in streamlining your processes with automatic data capture and leaving competitors in your wake, get in touch with Xtracta today. Our team of experts will discuss the process of integrating Xtracta technology with your existing software.