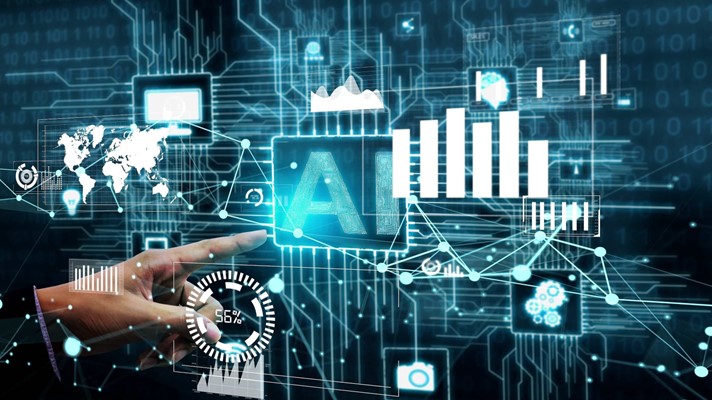
OCR, data extraction, and AI are common terms in today’s media, but the distinction between them is often blurred (e.g., OCR AI and OCR data extraction). So, what does OCR mean and how is it related to AI and data extraction? Below, we’ll clarify these terms for you. Read on for a quick overview of each and what sets them apart. Learn what makes these terms more than just buzzwords, how they should be used, and how they relate to each other within the context of Xtracta’s automatic data capture technology.
Optical Character Recognition (OCR)
Optical character recognition (OCR) lets you convert scanned images into textual documents that data can be extracted from. While digital data files (e.g., Microsoft Word documents) already contain digital data, scanned or photographed documents are just pixels that need to be converted to digital data before the text can be captured from them.
Whatever the format (scanned PDF, photograph, etc.), OCR can identify letters, numbers, and other characters within pixels in an image. Optical character recognition identifies the pixels that look like characters and words and then creates digital words and characters from them.
OCR technology is not always necessary before data extraction. As mentioned above, some PDFs will already be digital, such as those produced by an electronic system. For scanned documents, photos, and some old types of systems that still rasterise PDFs (convert them into pixels), optical character recognition is a necessary process for converting text to digital data.
Historically, most documents were scanned or faxed, which created a greater need for OCR. Today, more documents are created and distributed digitally (e.g., digital PDFs), meaning textual data is already present. In these cases, OCR is not typically needed. However, our experience at Xtracta has suggested that even some digital files will still contain pixelated data such as letterheads or logos. They still need to go through an OCR process to turn those into digital data.
Data Extraction
Data extraction is the process of finding specific pieces of data from a digital document. For example, if you have a passport scan and want to find the person’s date of birth, you need to find that data within the document. In the case of passports, OCR is often necessary because they are almost always scanned or photographed. Therefore, the pixels in the passport photo will need to be converted into digital data and then go through data extraction. After the pixels have been converted via OCR, data extraction can find the label (‘Date of Birth’, ‘D.O.B.’ and foreign language equivalents) and grab the data next to or underneath it.
A document that doesn’t require OCR can just go straight through data extraction. For example, a digital PDF that has been produced through printing in Microsoft Word will already have the digital text layer because it was produced from a digital file. Therefore, it’s not necessary to go through OCR because the textual data is already available for it.
Artificial Intelligence (AI)
Finally, Artificial intelligence (AI) applies broadly to both OCR and data extraction. Through AI and tools like machine learning (a subfield within artificial intelligence), models can be built to recognise certain types of patterns. These models are what power the OCR or data extraction process (using specific models for each).
A lot of state-of-the-art OCR technology now uses a type of AI called ‘deep learning’. Deep learning produces models that are very effective at converting pixels into data. Historically, things like handwriting were difficult to convert with OCR because it is so variable; traditional approaches to OCR couldn’t handle this. Today, deep learning-based models have improved OCR dramatically, to the extent that even handwriting can be converted into digital data with very high accuracy.
AI can also be applied to data extraction. For example, deep learning models can be used to learn document layouts and the relationships between labels (such as D.O.B) and text (the specific date). Through deep learning, the models can distinguish the types of data that people want to extract to narrow down your scope or validate other methods of comprehending candidates available for different data fields based on their content.
For example, let’s further explore the idea of training models to recognise the date of birth within documents. If you trained the model using a hundred different documents with date-of-birth variations among them, the system would see that every time you search for the date of birth, the information is in the form of a date. Through this, machine learning models can instantly improve the data extraction process because it knows that the corresponding information should be a date.
Essentially, machine learning discovers patterns in your data and makes decisions and recommendations based on data-driven analysis. This removes the need to build complex configurations for many scenarios.
Explore the advantages of OCR data capture technology for your organisation
Xtracta’s specialised approach to machine learning and intelligent document processing allows companies to maximise their workflow efficiency and accuracy. Get in touch with the team today to learn more about our receipt, contract, and invoice OCR software for your organisation.