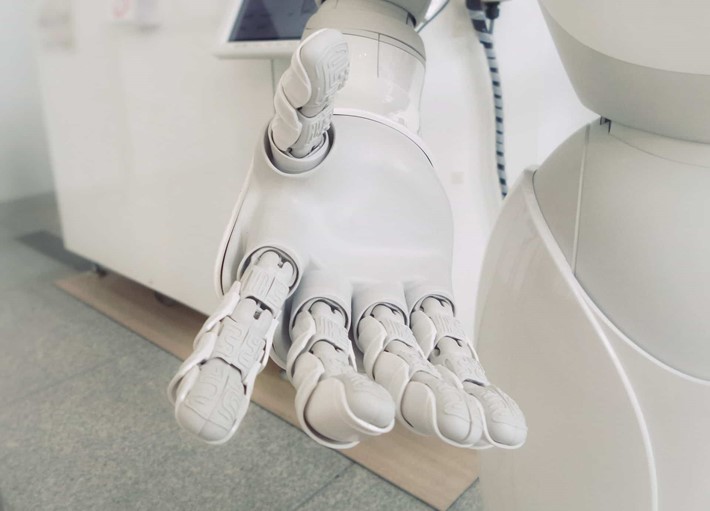
Recently, there has been a push towards deep learning and the opportunities it offers within the realm of artificial intelligence (AI). From self-driving cars to voice and image recognition, deep learning stands behind many technological innovations and already impacts multiple aspects of human life.
Today, we break down the fundamentals of deep machine learning, exploring its relevance within document data extraction software and explaining why—for Xtracta—it may not always be the best approach for our software or clients.
A Definition of Deep Learning
Deep learning is a class of machine learning algorithms that uses a multi-layered filter system to emulate the neural network and function of the human brain. By using thousands and thousands of interconnected processing nodes, deep learning enables systems to process, handle, and learn large volumes of data.
Deep learning technology is modelled on artificial neural networks (ANNs). These artificial neural networks are constantly receiving learning algorithms and expanding amounts of data to improve the performance of training processes (a.k.a. deep learning). Essentially, the more data a network has, the more efficient and accurate the training process becomes.
The training process is known as “deep learning” because over time the system’s neural network continues to expand, covering an ever-growing number of levels—the ‘deeper’ the level, the higher the productivity.
How Machine Deep Learning Works
There are two main phases within a machine deep learning process—training and inferring. During the training phase, large amounts of data are introduced to the system and matching characteristics are defined. Through comparing these characteristics, the system memorises them to refer to in the future when confronted with similar data.
In the inferring phase of the process, a deep learning AI uses the knowledge gained during the training phase to make conclusions and label new data it’s introduced to. In this way, a deep learning AI can make correct conclusions based on the characteristics of previously learned data.
The Advantages and Difficulties of Deep Learning
What are the main advantages of deep learning software?
- Its ability to create new functions from previously inferred features in the training dataset.
- High-level performance and accuracy.
- Its advanced analysis capabilities allow systems to learn and become smarter on their own.
What are the main difficulties of deep learning software?
- It requires continuous input data management and time-consuming initial implementation.
- Ensuring conclusion transparency is difficult because deep learning software is incapable of showing how it reached a certain conclusion.
- It is quite a resource-demanding technology (e.g., requiring powerful GPUs, large storage, etc.).
Machine Learning and Deep Learning: What’s the Difference?
Deep learning is in fact considered a part of the greater realm of machine learning. However in modern vernacular, the two are often confused as distinctly different ways of approaching AI.
Machine Learning
A subcategory of artificial intelligence (AI), machine learning is an approach driven by the idea that a computer can learn how to solve problems by itself, without relying on prewritten algorithms. Through a principle called supervised learning, users provide a machine with both training data and examples to help the system make decisions. There is also a principle called unsupervised learning where the machine attempts to group its own datasets to be used for subsequent training – while making it easier to procure and prepare training data, this approach tends to have inferior results to supervised learning.
In machine learning, the objective is to predict results based on the entered data. The more varied the entered data is, the easier it will be for the machine to determine patterns and produce accurate results.
While a machine learning system can solve many problems, it cannot create new tasks without input from a human.
Deep Learning
A branch of machine learning algorithms, deep learning is an approach that relies upon a multi-layered system emulating brains via a concept known as “neural networks” to extract useful data. Each input layer—following in hierarchical order—receives the data output of the previous layer, emulating the synaptic neural network of a human brain. A deep learning system that relies on an artificial neural network will act and make decisions based on its own experience—thus, emulating the way a human brain works.
One of the fundamental differences between deep learning and non-deep learning machining learning approaches is that a deep learning system can formulate new knowledge and conclusions based on the large amount of data loaded into the machine – i.e. it tends to be less confined in how it operates and what it can deduce. Compared to more traditional machine learning approaches, deep learning requires much more time and data to train, as the user needs to label and input a lot more training data into a machine so that it can make accurate conclusions.
How does deep learning affect intelligent document processing?
There are many different types of machine learning and each approach has an impact on the way an extraction model should be trained, and how it will perform. While deep learning is now growing in popularity, when it comes to incorporating a deep learning approach into intelligent document processing—it may not be the best technological choice.
This is because a deep learning approach to train a system requires an immense amount of data, high-performance hardware, and training time. For example, during the early stages of the model training, a mass amount of labelled training data needs to be implemented to enable the machine to formulate decisions and a significant amount of processing time and power is also required to determine a response. For many Xtracta clients, this would mean considerably more effort to get up and running with deep learning models compared to some of the other approaches Xtracta takes to minimise the time required to prepare training data.
Xtracta does use deep learning approaches in-part where it has made the effort to produce the required training data itself. This results in a greater hybrid approach where users get many of the advantages of deep learning for IDP applications while still keeping training data volumes (and thus effort/implementation cost) low.
Talk to Xtracta today to discover the benefits of automated data entry software!
Through its specialised approach to machine learning and intelligent document processing software, the Xtracta engine is able to offer clients maximised workflow efficiency and incredible document classification accuracy without the time-consuming and costly nature of implementation. By taking a hybrid approach to deep learning and traditional machine learning with Xtracta, the time to onboard businesses, train them, and get started is also significantly less costly.
Talk to the team at Xtracta today to learn more about our AI-powered data extraction software and discover the benefits of integrating it into your own software.