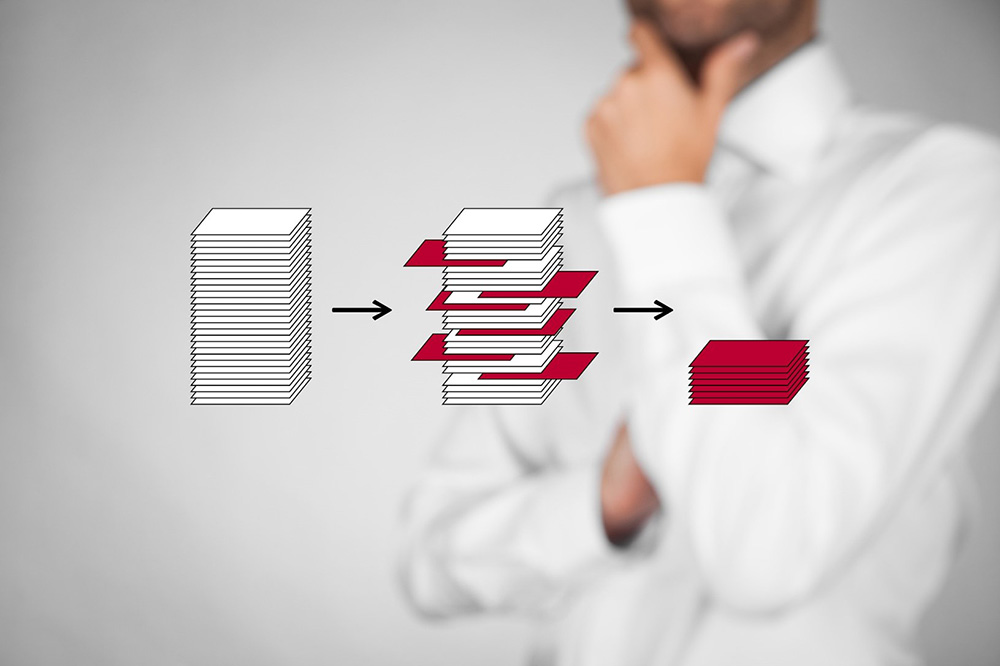
When it comes to fast and efficient document processing, how beneficial is it to have automation technology to process data? Does intelligent document processing (IDP) apply to unstructured data? We’ll explore these questions below, elaborating on the difference between structured and unstructured data and why it’s important to have data capture technology that can process and classify documents.
What is Unstructured Data?
Unstructured data is the most abundant form of big data and can come in textual and non-textual formats. It is so abundant because of its diverse nature; it could be anything from text data, media, and images to audio streams, social media posts, sensor data, and more.
Unstructured data is so-called because it isn’t in a format typically compatible with a relational database. It is comprised of datasets (typically large collections of files) with an internal structure that is not predefined through data models. It could be human-made or machine-generated.
Due to the increased number of digital applications and services today, unstructured data is on the rise, estimated by some to make up 80-90% of the digital data universe.
While structured data is essential, unstructured data can be very valuable if utilised correctly, as it can provide a wealth of information and insights that would otherwise remain unseen.
The difference between Structured, Semi-Structured and Unstructured Data
Structured, unstructured, and semi-structured data can all exist within the category of “big data.” All three types of data can be utilised to gain valuable company insights, but it is important to know which data to collect and analyse for the insights you’re looking for. It is also worth noting that most intelligent document processing use-cases relate only to structured or semi-structured data. Most use-cases of gaining insights into unstructured data may relate to areas such as sentiment analysis which is separate and unique from IDP.
Structured Data
Key features typical of structured document data include:
- Same datapoints
- Consistent formatting/layout
- Stored in a structured framework defined by pre-set parameters
Structured data is consistent and resides in pre-defined formats. Typically, structured data is mapped in a structured framework of columns/rows and can be both quantitative and qualitative. Structured data is designed for easy processing (data entry, search, comparison, and extraction) and analysing, and can be sourced both automatically and manually depending on the data model. A good example of structured document data is a government form that follows a template and that asks for details like name, date of birth, etc. with exactly the same data fields and layout on each copy of the form but with each copy having the individual filling the forms unique details, relevant for each field of data.
Unstructured Data
Key features typical of unstructured document data include:
- Text-heavy
- Not organized in a predefined format or model
- It may be organised by theme or subject but not specific data elements
While unstructured data includes statistics, facts, and figures, the text is often dense and configured in a way that is challenging to sort through, extract, and organise. For example, social media posts can include everything from a range of topics being discussed to different opinions, and recommendations—all of which need to be organised and analysed to gain insights.
Semi-Structured Data
Key features of semi-structured document data include:
- Similar/same data fields but usually structured/laid out in different ways
- Some repetition of structures and data layout
- Includes all kinds of documents in many areas such as legal, accounting, logistics, and many more
Semi-structured document data lies in between structured and unstructured data although it is more akin to structured data than unstructured. This type of data has similarities with unstructured data in that there are a plethora of structures in which the data could be laid out in. However, the actual data that each document contains is usually of consistent types like in structured data, so it can be transposed into specifically desired labels or fields. For example, two contracts can look and read very differently when drafted by different people. However, they would almost certainly contain the same fields, such as the date of the contract, parties entering the agreement, and so on.
With the advent of IDP technology, there is less cost to capture semi-structured data with data that may not necessarily be used for business processing also able to be captured at no additional cost, enabling better insights. For example, in invoice extraction, companies may have only previously been manually entering an invoice total. With IDP, they can now capture all the items being purchased with no additional work or cost. This extra information can give them more granular accounting information and spend analysis. Most IDP use cases today are focussed on semi-structured document data extraction.
Why is it important to have data capture technology that can process and classify all types of documents?
While structured data is important, over 80% of valuable enterprise data is not structured. Without effective data capture technology, the valuable information and insights that can be gleaned from this data remain untapped.
Businesses need to find a way to manage and obtain both insights from their unstructured data (as this information can play an important role in business decision-making) alongside operational processing of data found in both structured and semi-structured documents. Gaining insights into unstructured data (and increasingly semi-structured data) can help them improve their services and customer satisfaction while further reducing unnecessary expenditures and giving them a competitive edge over rival companies. Efficient automation of the processing of structured and semi-structured data can help them reduce processing costs and increase their responsiveness.
The advantage of AI-powered data extraction
When it comes to effective data capture technology, Xtracta’s OCR-powered data extraction technology is centred around advanced artificial intelligence (AI) and can automatically process and decipher correct information from virtually any document format—both structured and semi-structured.
Through machine learning, our data capture technology will learn new document types as it encounters them, processing them with far greater speed and accuracy than manual data entry workers. Rather than spending hours sorting through documents manually, your staff will typically only need to review a small number of documents, which usually reduces over time as the machine learning and AI models improve. This will considerably reduce the amount of time spent on repetitive paperwork, saving your staff hours of valuable time for more rewarding tasks.
If you’re looking for a receipt, invoice, and contract capture API technology that can configure any number of document types, get in touch with the team at Xtracta. Discover how Xtracta’s invoice scanning and data capture software can revolutionise your business, reduce costs, and free up resources today.