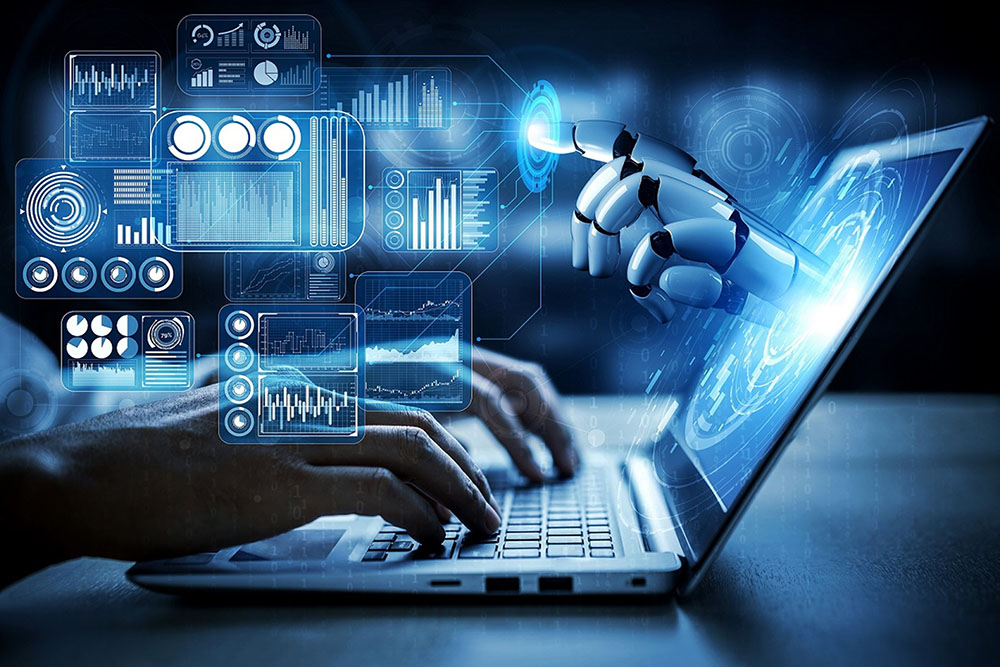
While there are countless companies providing data extraction software, most of them use template-based software, requiring users to input a document map first. In this blog post, we discuss why Xtracta is different, and how machine learning allows our software to discover, classify, and understand new and complex documents with far greater efficiency than template-based processing software.
To establish how Xtracta’s machine learning approach is different from other data capture models, let’s briefly explore traditional forms of data capture and template-based processing.
Traditional Forms of Data Capture
Before the days of OCR data capture, documents such as invoices and contracts were once processed manually by data entry clerks, who would spend hours copying information from paper documents to input into an IT system by hand. This was a slow and monotonous process that was prone to errors, and not scalable at all.
The Rise of Template-Based Processing
Following this origin of manual data entry came the invention of optical character recognition (OCR) technology. OCR brought about the first commercial solutions for automated text recognition and with it, an easier way for businesses to process information.
By implementing a “rule-based” approach to data processing that relied upon an OCR template structure, users could create zonal templates that extracted text from scanned images. These templates allowed a computer system to isolate certain sections of text, at specific locations in the page, and read it in a set way. For example, if you wanted to extract a date or address within a document using a template format, separate configurations would need to be created, and the system would need to be manually configured with where to find the specific field in the document.
Through this rule-based approach, computers could generate searchable text from an image without the need to manually input every letter and word.
From this, we can see that template-based OCR was able to speed up some elements of data processing. However, this process still had its limitations.
Limits of Template-Based Data Capture
While template-based data capture is still used today, there are common challenges the technology is faced with, such as dealing with semi-structured or unstructured data.
Semi structured Data
For semi-structured documents such as invoices, template-based software can offer a workable solution if templates are created for each of the different semi-structured designs. The downside of this approach is that it’s quite labour-intensive to generate templates to cater to each variation in design, and companies need employees to be trained and experienced in template creation to do this. Often, businesses need a trained operator or specialist to create these templates.
This means that a large amount of time needs to be dedicated to setup. It also means that as time progresses, it’s likely that those templates will need to be modified or replaced to suit changes in formats sent within the company, or sent by trading partners such as suppliers (with documents such as invoices, statements etc.) or customers (with documents such as purchase orders, remittances, etc.).
Fully Unstructured Data
Data such as hand-written notes, emails, blog posts, videos, and digital images are typical examples of unstructured data. While emails and binary file formats may have meta-data and well-defined headers, the content itself is fully unstructured.
To process a text-heavy document with no clear layout, a processing system needs to find the information from the unstructured text and extract structure (i.e., context) from it. For template-based processing software, there is really no possibility of this because it relies on pre-set template structure.
The Role of Machine Learning in Xtracta
Compared to template-based processing, Xtracta uses a machine learning approach to data extraction. Through machine learning, Xtracta’s API can process countless documents in virtually all forms of data including formats such as semi-structured and unstructured data.
The Benefit of a Trainable Model Approach to Data Capture
Rather than using a layout-based template, Xtracta uses trainable models that can find the information users want. As Xtracta is powered by artificial intelligence and machine learning technology, the document classification software constantly learns from user interaction and each new document processed. This self-learning ability of Xtracta is what enables hands-free data extraction to take place, increasing result accuracy and minimising the need for human management.
Over time, the more data that is put into the system, the more accurate and self-sufficient the API becomes. It’s also very simple for a standard user to train a model. When viewing a scanned document, all the user needs to do is click on the text that they want to copy for each field, and it will automatically be filled.
For example, if a user is training the AI to capture the address and information of job applicants from resumes, all they need to do is put the cursor into the address field and the information will be filled.
In this way, users can easily train the model to learn and capture data from different fields. Additionally, because of its intuitive design, no specialist skills are required to train the models.
Based on the training, Xtracta can build multiple types of models for each document design it processes, and it also has a much greater capacity to handle deviations inside of documents—which template layouts simply can’t accommodate for.
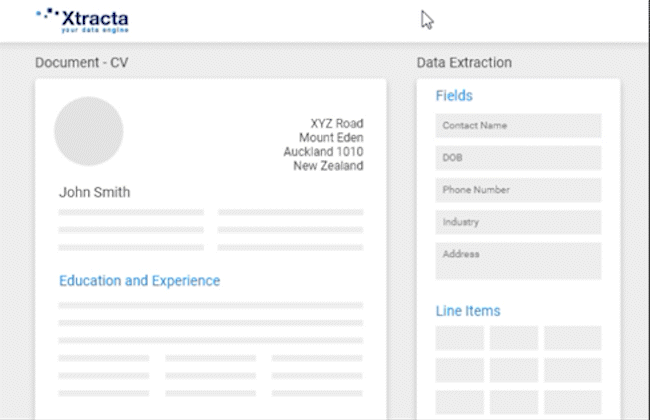
Using Xtracta to Create General Models
The Xtracta engine can also create ‘general models’ that can be applied to virtually any document, no matter the layout or design variation.
For example, if a user wants to create a general model to capture all the educational backgrounds of job applicants, all they need to do is select the schools/universities of applicants on their CVs to teach the engine.
As the engine is trained to recognise these features in many CVs of varying formats, it will start recognising consistencies across every sample (such as the terminology, e.g., ‘university’, ‘bachelors’, ‘diploma’, etc., and section titles, e.g., ‘education’, ‘qualifications’). From this, it can generate a model that can be applied to virtually any CV type to extract highly accurate results.
Talk to Xtracta today about implementing data capture technology in your company
Regardless of the industry, Xtracta’s data capture technology can be configured to efficiently process any number of document types, regardless of structure. Talk to our team of specialists to discover how Xtracta can be implemented to reduce costs, increase accuracy, and revolutionise your company today.